The Future of Data Science in Healthcare: Revolutionizing Medicine
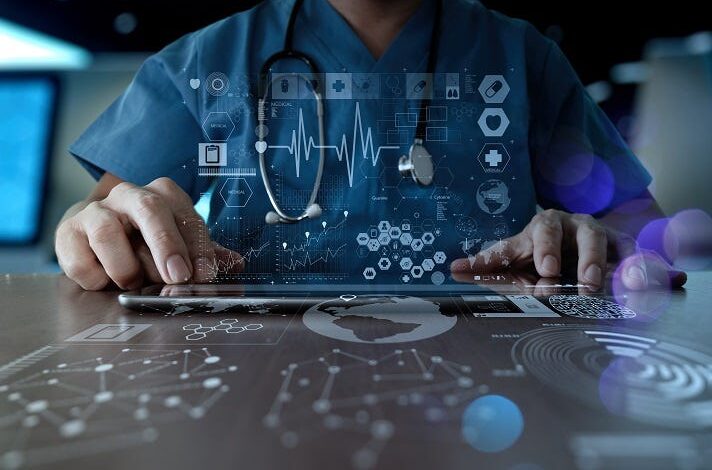
In recent years, data science has emerged as a transformative force in various industries, with healthcare being one of the most promising fields. The convergence of data science and healthcare holds immense potential for improving patient outcomes, optimizing operational efficiency, and driving innovation. As we look to the future, several key trends and advancements are set to redefine the landscape of healthcare through the lens of data science.
1. Predictive Analytics and Personalized Medicine
One of the most exciting prospects of data science in healthcare is the ability to predict and prevent diseases before they manifest. Predictive analytics leverages vast amounts of patient data, including medical history, genetic information, and lifestyle factors, to identify patterns and risk factors associated with various conditions. This enables healthcare providers to implement personalized prevention strategies, tailor treatments to individual patients, and reduce the incidence of chronic diseases .
Personalized medicine, also known as precision medicine, is another area where data science is making significant strides. By analyzing genetic data and other biomarkers, scientists can develop targeted therapies that are more effective and have fewer side effects compared to traditional treatments. This approach not only enhances patient outcomes but also paves the way for breakthroughs in treating complex diseases like cancer .
2. Enhanced Diagnostics through Machine Learning
Machine learning algorithms are revolutionizing diagnostics by enabling faster, more accurate, and less invasive methods of detecting diseases. These algorithms can analyze medical images, such as X-rays, MRIs, and CT scans, with remarkable precision, often surpassing human radiologists in identifying abnormalities. Additionally, machine learning models can sift through electronic health records (EHRs) to identify patterns that may indicate the early stages of diseases, leading to timely interventions .
For instance, Google’s DeepMind has developed AI models capable of diagnosing eye diseases from retinal scans with an accuracy comparable to that of expert ophthalmologists. Similarly, AI-powered tools are being used to detect skin cancers, cardiovascular diseases, and even mental health disorders through the analysis of various data sources .
3. Streamlining Operations and Reducing Costs
Data science is also playing a crucial role in optimizing healthcare operations and reducing costs. Predictive analytics can help hospitals manage resources more efficiently by forecasting patient admissions, bed occupancy rates, and staffing needs. This ensures that healthcare facilities are adequately prepared to handle patient influxes, especially during peak times or emergencies .
Moreover, data-driven insights can streamline supply chain management, reduce waste, and enhance the overall efficiency of healthcare systems. By identifying inefficiencies and areas for improvement, healthcare providers can allocate resources more effectively and reduce operational costs, ultimately benefiting both patients and providers .
4. Improving Patient Engagement and Experience
The integration of data science into healthcare is transforming the way patients interact with the healthcare system. Wearable devices and mobile health apps collect real-time data on various health metrics, such as heart rate, physical activity, and sleep patterns. This data can be used to provide personalized health recommendations, monitor chronic conditions, and alert healthcare providers to potential issues before they become critical .
Telemedicine, which gained significant traction during the COVID-19 pandemic, is another area where data science is enhancing patient experience. By leveraging data analytics, telemedicine platforms can offer more personalized and efficient care, ensuring that patients receive the right treatment at the right time, regardless of their location .
5. Advancing Medical Research
Data science is revolutionizing medical research by enabling the analysis of massive datasets to uncover new insights into diseases, treatments, and healthcare practices. The use of big data analytics allows researchers to identify correlations and causal relationships that were previously impossible to detect. This accelerates the pace of discovery and innovation, leading to the development of new therapies and medical technologies .
For example, the analysis of genomic data has led to significant advancements in understanding the genetic basis of diseases and the development of gene therapies. Similarly, data-driven research is driving the development of personalized vaccines, regenerative medicine, and other cutting-edge treatments that have the potential to transform healthcare .
Challenges and Considerations
While the future of data science in healthcare is undoubtedly promising, it is not without challenges. Data privacy and security are paramount concerns, as the sensitive nature of health data makes it a prime target for cyberattacks. Ensuring the ethical use of data and maintaining patient confidentiality must be at the forefront of any data science initiative in healthcare .
Additionally, the integration of data science into healthcare requires significant investment in infrastructure, technology, and training. Healthcare providers must be equipped with the necessary tools and skills to leverage data effectively, and there must be a concerted effort to bridge the gap between data scientists and healthcare professionals .
Conclusion
The future of data science in healthcare is bright, with the potential to revolutionize every aspect of the industry, from diagnostics and treatment to operations and patient engagement. By harnessing the power of data, healthcare providers can deliver more personalized, efficient, and effective care, ultimately improving patient outcomes and advancing medical research. As we move forward, it is essential to address the challenges and ethical considerations associated with data science to ensure that its benefits are realized in a responsible and sustainable manner.
References
- Khamsi, R. (2021). How AI-powered precision medicine will transform healthcare. Nature.
- Topol, E. J. (2019). High-performance medicine: the convergence of human and artificial intelligence. Nature Medicine.
- Collins, F. S., & Varmus, H. (2015). A new initiative on precision medicine. New England Journal of Medicine.
- Esteva, A., et al. (2017). Dermatologist-level classification of skin cancer with deep neural networks. Nature.
- De Fauw, J., et al. (2018). Clinically applicable deep learning for diagnosis and referral in retinal disease. Nature Medicine.
- Reddy, S., Fox, J., & Purohit, M. P. (2019). Artificial intelligence-enabled healthcare delivery. Journal of the Royal Society of Medicine.
- Bates, D. W., et al. (2018). Big data in health care: using analytics to identify and manage high-risk and high-cost patients. Health Affairs.
- Steinhubl, S. R., Muse, E. D., & Topol, E. J. (2015). Can mobile health technologies transform health care? JAMA.
- Keesara, S., Jonas, A., & Schulman, K. (2020). Covid-19 and health care’s digital revolution. New England Journal of Medicine.
- Wang, Z., et al. (2018). Artificial intelligence in healthcare: past, present and future. Stroke and Vascular Neurology.
- Ashley, E. A. (2016). Towards precision medicine. Nature Reviews Genetics.
- Rieke, N., et al. (2020). The future of digital health with federated learning. npj Digital Medicine.
- Parikh, R. B., Teeple, S., & Navathe, A. S. (2019). Addressing bias in artificial intelligence in health care. JAMA Network Open.
For More: Data Science
Course: Data Science